What constitutes cost in AI projects
In the rapidly evolving digital landscape, artificial intelligence has emerged as a transformative force, reshaping industries and redefining the way businesses operate. From automating routine tasks to generating insights from vast amounts of data, AI offers a wealth of opportunities for businesses to increase efficiency, improve decision-making, and create new value. However, the journey towards AI adoption is not without its challenges, and one of the most significant among these is understanding and managing the associated costs.
The cost of AI projects is a multifaceted concept that extends beyond the mere financial expenditure on technology or services. It encompasses a broad spectrum of direct and indirect expenses, investments in time and resources, and potential trade-offs that can impact a business's operations and strategic objectives.
Key cost elements in an AI project include:
- Talent Acquisition and Training: Skilled professionals, such as data scientists and AI specialists, are crucial for the successful execution of AI projects. The costs of hiring, training, and retaining these professionals can be substantial.
- Data Acquisition and Preparation: AI systems rely on data to learn and make decisions. The process of acquiring, cleaning, and preparing this data for use can be a significant cost factor.
- Infrastructure: AI projects often require robust computational resources and specific hardware or software. Some projects also require specialized hardware like GPUs to scale better. The cost of procuring, maintaining, and upgrading this infrastructure should be factored into the overall project cost.
- Development and Implementation: This includes the expenses associated with the design and development of AI models, as well as their integration into existing systems.
- Maintenance and Upgrades: AI systems need regular maintenance and updates to remain effective. These ongoing costs can accumulate over the lifespan of the project.
- Regulatory Compliance and Risk Mitigation: Depending on the industry and nature of the AI application, there may be costs related to regulatory compliance, data privacy, and security. Additionally, businesses should account for potential risks and the costs of mitigating them.
Understanding these cost elements is the first step towards effective financial planning for AI projects. However, it's equally important to recognize that the cost of AI adoption is not just an expenditure; it's an investment. The value derived from AI - whether in the form of increased efficiency, improved decision-making, or innovative new products and services - can far outweigh the initial and ongoing costs.
Therefore, when considering the cost of AI projects, business decision-makers should adopt a holistic total cost of ownership perspective (TCO) that balances the financial implications with the strategic benefits. This involves not only a thorough understanding of the cost elements but also an assessment of the potential return on investment, the alignment with business objectives, and the capacity for future growth and innovation.
In the end, the goal of understanding the cost of AI projects is not merely to minimize expenditure and to remain competitive. By making informed decisions about where and how to invest in AI, businesses can ensure that they are not just spending wisely, but also positioning themselves for success in the AI-driven future.
Typical approaches to AI projects
As a business decision-maker, when you embark on the journey of AI adoption, you are presented with a variety of choices. Each choice represents a different approach to implementing AI, and each comes with its own set of costs, benefits, and considerations. Understanding these choices is crucial for making an informed decision that aligns with your business's needs, goals, and resources. Here are the primary choices you have:
The Team Building Approach
This approach involves building an in-house team of AI specialists, such as data scientists, machine learning engineers, and data engineers, to develop and implement AI solutions. This approach offers a high degree of control and customization, allowing you to tailor AI solutions to your specific needs. However, it also requires significant investment in talent acquisition, training, and retention.
- Cost Elements: Building an in-house team involves costs such as hiring and retaining skilled professionals, investing in necessary hardware and software, and ongoing training and development. There may also be costs associated with creating a suitable work environment for the team, including office space and equipment.
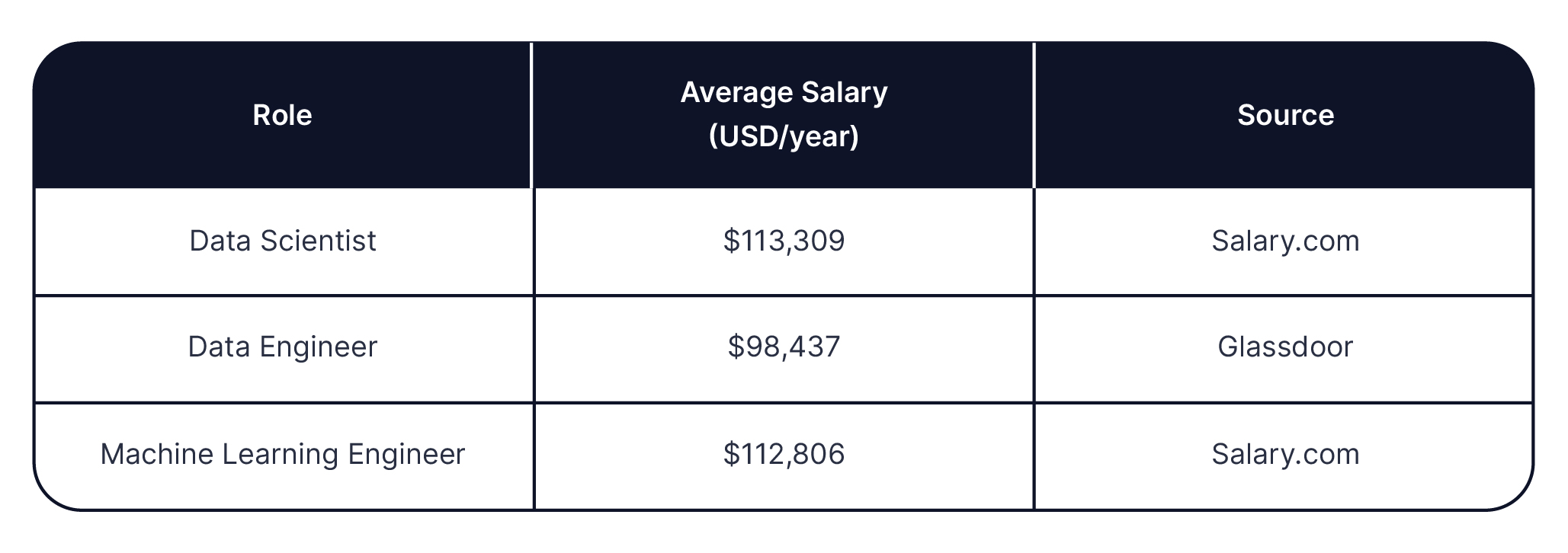
- Time to Value: The time to value can be longer with this approach, as it involves recruiting the right talent, setting up the necessary infrastructure, and developing the AI solution from scratch. And finding the talent with the domain expertise needed for your business and to build the solutions for your use cases is even harder. However, once the team is in place and the initial development is done, the time to value for subsequent projects may be shorter.
- Challenges to Anticipate: Challenges can include finding and retaining the right talent, managing the team effectively, staying up-to-date with the latest AI technologies and techniques, and integrating the AI solutions with existing systems and processes.
The Cloud Provider Approach
Many cloud providers offer AI services that you can use on a pay-as-you-go basis. These services can range from pre-trained AI models to machine learning platforms that allow you to train your own models. This approach offers scalability and flexibility, but it also requires ongoing subscription fees and can lead to vendor lock-in.
- Cost Elements: The primary costs with this approach are the subscription fees for the cloud services, which are typically based on usage. There may also be costs for data storage and transfer, as well as for any necessary hardware or software. It is worth noting that the cost of cloud provider approach is always in addition to the cost of building your own team.
For the purpose of this estimation, we will assume the following:
- The AI model will be trained once a week and predictions will be made once a day.
- The model will be trained on a machine with 2 vCPUs and 8 GiB RAM.
- The data will be stored in the cloud provider's storage service.
- The total data size is 10 GB.
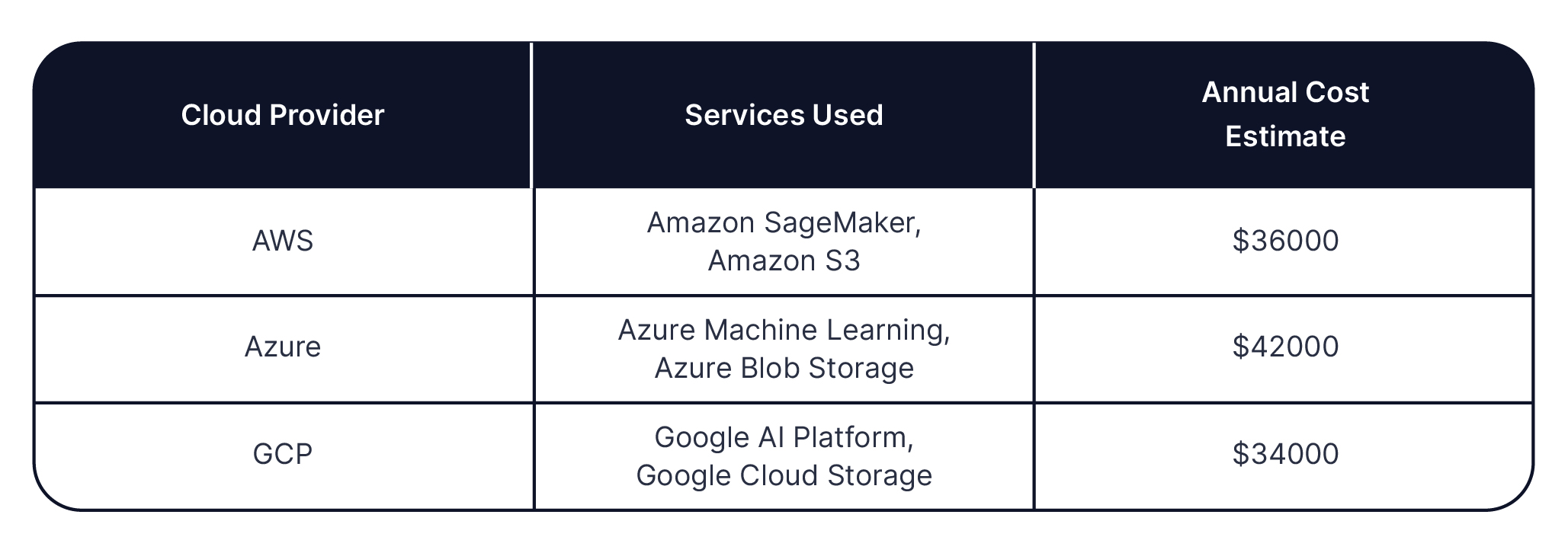
The cost of cloud services is always in addition to the cost of personnel.
- Time to Value: The time to value can be relatively short with this approach, as the cloud provider typically offers pre-built AI services that can be used immediately. However, customization and integration with existing systems can add to the time to value.
- Challenges to Anticipate: Challenges can include vendor lock-in, data security and privacy concerns, potential limitations of the pre-built AI services, limitations with integrating existing systems, and managing costs as usage increases.
The AI Service Provider Approach
AI service providers are companies that specialize in developing and implementing AI solutions for other businesses. This approach allows you to leverage the expertise of specialists without having to build your own team. However, it can also lead to less control over your AI solutions and potential communication and coordination challenges.
- Cost Elements: The primary costs with this approach are the fees for the AI services, which can be based on a variety of factors such as the complexity of the project, the level of customization required, and the duration of the engagement.
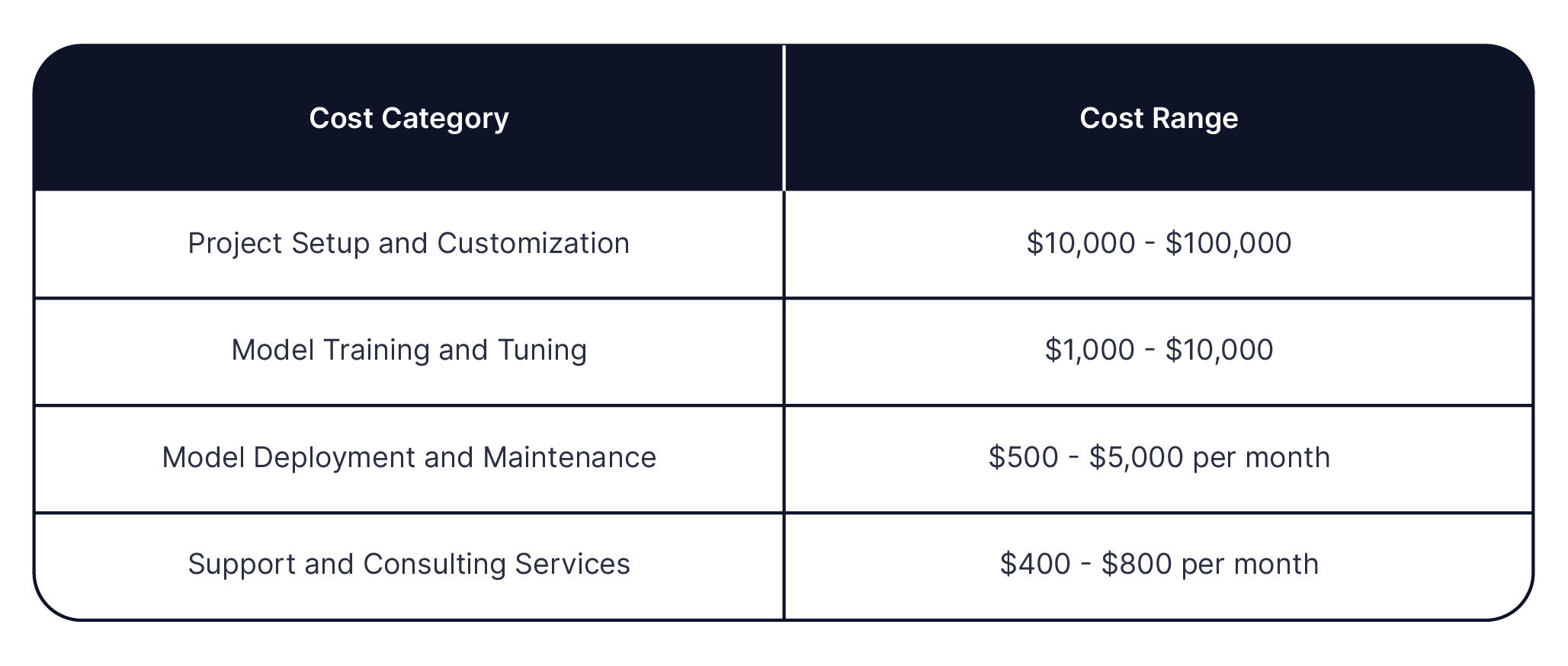
Costs can vary greatly depending on geography of the providers, rate cards for resources deployed, complexity of requirements and change logs.
- Time to Value: The time to value can vary widely with this approach, depending on the specifics of the project and the capabilities of the service provider. However, since the service provider brings expertise and experience, the time to value can often be shorter than with the team building approach.
- Challenges to Anticipate: Challenges can include communication and coordination with the service provider, less control over the AI solutions, and potential difficulties in integrating the solutions with existing systems and processes.
The AI Platform Approach
AI platforms are comprehensive solutions that provide a range of tools and services for developing, training, deploying, and managing AI models. These platforms can offer a balance of control and convenience, allowing you to customize your AI solutions while also benefiting from built-in tools and services. However, they can also require significant investment and technical expertise.
- Cost Elements: The primary costs with this approach are the fees for the AI platform, which can include upfront costs for licenses or subscriptions, as well as ongoing costs for updates and support. There may also be costs for training staff to use the platform effectively.
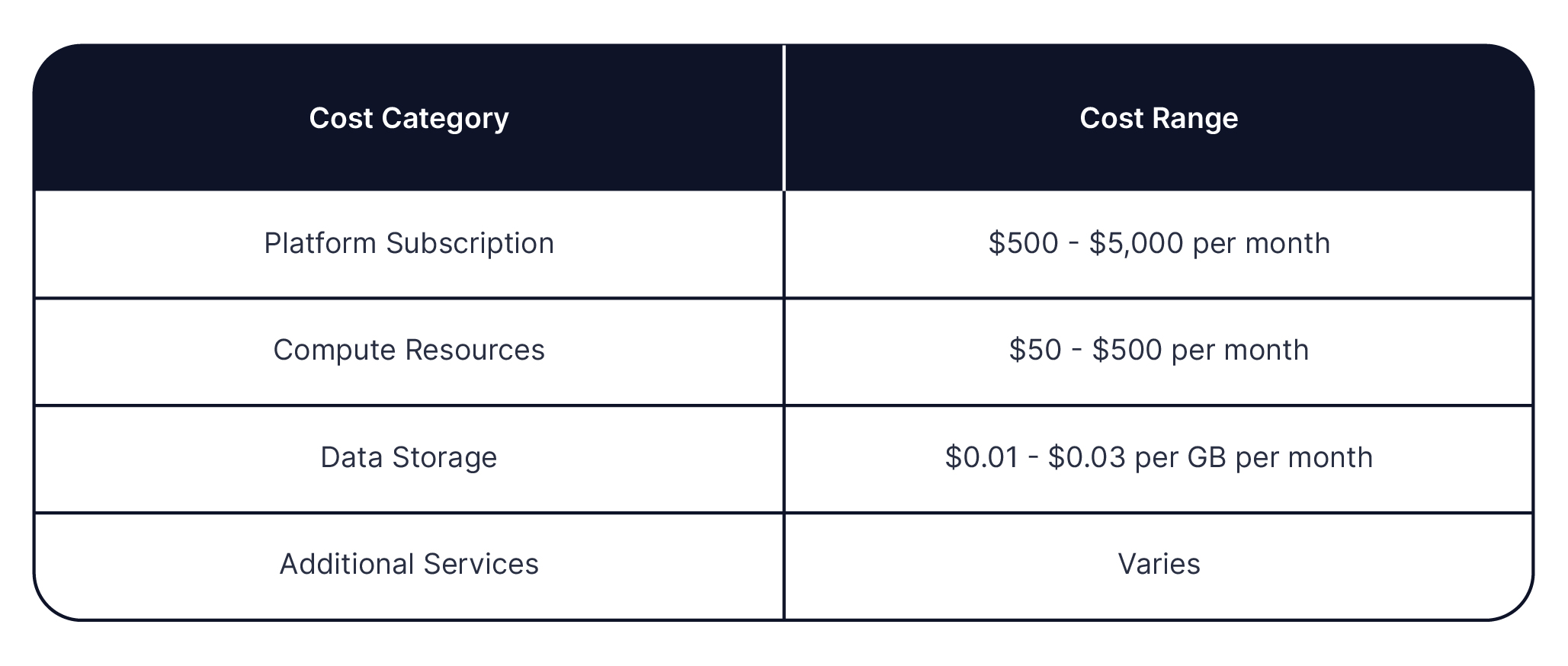
Cost tends to be stable and proportional to the scale of utilisation.
- Time to Value: The time to value can be relatively short with this approach, as the platform provides a range of built-in tools and services that can accelerate the development and deployment of AI solutions. However, customization and integration can add to the time to value.
- Challenges to Anticipate: Challenges can include learning to use the platform effectively, potential limitations of the platform's capabilities, and managing costs as usage of the platform increases.
Each of these choices represents a different balance of cost, control, customization, and convenience. The right choice for your business will depend on a variety of factors, including your budget, your technical capabilities, your specific needs, and your strategic goals. By understanding these choices and considering them in the context of your own circumstances, you can make an informed decision that will set your business up for success in its AI adoption journey.
Cost critical trade-offs
In the journey of AI adoption, decision-makers often encounter various trade-offs. Understanding these trade-offs is crucial for making informed decisions that align with the company's strategic goals and resources. Here are some of the most common trade-offs:
- Upfront vs. Ongoing Costs: Upfront costs, such as investment in hardware or initial setup fees, are one-time expenses that occur at the beginning of the project. Ongoing costs, such as subscription fees or maintenance costs, are recurring expenses that continue over the lifespan of the project. A solution with high upfront costs but low ongoing costs might be more cost-effective in the long run, but it requires a larger initial investment.
- Custom Development vs. Off-the-Shelf Solutions: Custom development offers a high degree of customization and control, allowing you to tailor the AI solution to your specific needs. However, it often involves higher costs and longer implementation times. Off-the-shelf solutions are typically quicker to implement and less expensive, but they may not offer the same level of customization or control.
- On-Premises vs. Cloud-Based Solutions: On-premises solutions offer more control over data and processes, but they often involve higher upfront costs for hardware and setup. Cloud-based solutions typically have lower upfront costs and offer greater scalability, but they come with ongoing subscription fees and potential concerns about data security and privacy.
- Pay-As-You-Go vs. Fixed Pricing: Pay-as-you-go pricing allows you to pay only for what you use, offering flexibility and scalability. However, it can lead to unpredictable costs if your usage increases significantly. Fixed pricing offers more predictability, but you might end up overpaying if you don't use the service to its full extent.
- Investing in Talent vs. Outsourcing: Building an in-house team offers more control and potentially better integration with existing systems and processes, but it involves costs for hiring, training, and retaining skilled employees. Outsourcing to an AI services company can provide access to expertise and reduce management overhead, but it might result in less control and potential difficulties with communication and coordination.
- Short-Term vs. Long-Term Costs and Benefits: Some costs, like setup and integration, are mostly short-term, while others, like maintenance and updates, are long-term. Similarly, some benefits, like process automation, might be realized quickly, while others, like data-driven decision making, might take longer to materialize. Balancing these short-term and long-term costs and benefits is crucial for making a decision that's right for your company's current situation and future plans.
Each of these trade-offs presents a different balance of cost, risk, and benefit. The right balance for your business will depend on a variety of factors, including your budget, your technical capabilities, your specific needs, and your strategic goals. By understanding these trade-offs and considering them in the context of your own circumstances, you can make an informed decision that will set your business up for success in its AI adoption journey.
How should you decide on your approach
Choosing the right approach for AI adoption is a strategic decision that can significantly impact your business's operations, capabilities, and future growth. Here is a framework to guide you through this decision-making process:
- Use Principle of Proportion: Align the scale and complexity of your AI project with your business size, needs, and resources. A small to mid size business with limited resources might benefit more from an AI Platform approach since that enables experimentation and see value before you scale, while a large corporation with substantial resources might opt for building an in-house team or using an AI platform.
- Engage Early and Efficiently: Start planning for AI adoption as early as possible. This allows you to thoroughly assess your needs, explore different options, and make an informed decision. Engage efficiently by focusing on the options that best align with your business's strategic goals and resources.
- Discover What It Takes for Your AI Project: Understand the specific requirements of your AI project. This includes the type of AI technology you need (e.g., machine learning, natural language processing, computer vision), the data you have available, the skills and expertise required, and the integration with existing systems.
- Are You Future-Proofing from Tech Disruptions: Consider how your chosen approach will stand up to future technological advancements and changes in your business environment. A good AI adoption strategy should be flexible and adaptable, allowing you to take advantage of new AI technologies and techniques as they emerge.
- Make an Effective Choice: Finally, make your choice based on a comprehensive understanding of the costs, benefits, and trade-offs of each approach. This should not be a rushed decision, but rather a strategic one that considers both the short-term and long-term implications for your business.
This framework is not a one-size-fits-all solution, but rather a guide to help you navigate the complex cost considerations in your endeavor for AI adoption. By following this framework, you can make a decision that is not only cost-effective but also strategically aligned with your business's needs, goals, and resources.
Why RapidCanvas is always a smart choice
RapidCanvas is a no-code AutoAI Platform designed to streamline the process of creating and deploying AI solutions, making them an ideal starting point for businesses looking to leverage AI. The platform capabilities coupled with the team’s deep experience working with a wide range of AI solutions makes RapidCanvas a complete AI partner.
Creating an AI solution has never been easier, and all with simple and transparent pricing that covers all of the following:
- Cost of solution/model development, using a pre-built solution library
- Cost of platform subscription & licenses for all users
- Cost of maintenance
- Cost of support services
Want more reasons to get started with RapidCanvas?
- No Coding Required: RapidCanvas allows you to create AI solutions without any coding. This means that even non-technical team members can contribute to the AI development process, democratizing AI within your organization.
- Rapid Deployment: RapidCanvas enables you to go from idea to AI solution in as little as four weeks. This rapid deployment can give you a competitive edge by allowing you to start leveraging AI faster.
- Collaborative Environment: RapidCanvas provides a unified interface that facilitates collaboration across cross-functional teams. This can help to ensure that all stakeholders are aligned and working towards the same goals.
- Expert Support: With RapidCanvas, you gain access to a world-class team of experts who can help you navigate the complexities of AI development.
- Pre-configured Solutions: RapidCanvas offers pre-configured AI solutions for various use cases, which can be easily customized to meet your specific needs.
- Scalability: RapidCanvas is designed to scale with your business. Whether you're processing hundreds or millions of data points, RapidCanvas can handle it, ensuring that your AI solutions can grow with your business.
- Cost-effective: With RapidCanvas, you enable your teams to take advantage of AI solutions to see real business outcomes, without investments in new data science and engineering resources.