Changes fuelling AI adoption today
Artificial Intelligence (AI) has emerged as a transformative force in the realm of business technology. Among the various manifestations of AI, Large Language Models (LLMs) - such as OpenAI's GPT-3 and Google's BERT - have distinguished themselves as potent tools, fundamentally altering operational paradigms across organisations.
LLMs are sophisticated AI constructs capable of comprehending and generating text that closely mirrors human language. Their training involves the processing of extensive data volumes, enabling them to emulate human linguistic patterns and produce contextually appropriate responses. This proficiency in 'understanding' and 'communicating' in human language has broadened the scope of business applications.
Consider the following applications of LLMs in the commercial sphere:
- Customer Service: LLMs are employed to drive chatbots and virtual assistants, offering round-the-clock customer support and liberating human agents to address more intricate tasks.
- Content Generation: LLMs can generate a wide range of content, from product descriptions to marketing copy, delivering high-quality output at an industrial scale.
- Data Analysis: LLMs can navigate through vast quantities of text data, extracting valuable insights and identifying patterns that might elude human analysis.
The ascendance of LLMs is indicative of a more extensive trend of AI adoption across various industries. Businesses are progressively acknowledging the importance of AI, not merely as an instrument for automation, but as a strategic resource capable of driving growth and innovation.
However, the process of AI adoption is not homogeneous. It exhibits considerable variation across industries and individual organisations. While some businesses are at the vanguard, harnessing AI to secure a competitive advantage, others are at the nascent stages of their AI journey, exploring avenues to integrate AI into their operational framework.
Several factors underpin this trend of AI adoption:
- Technological Advancements: The swift pace of AI research and development has rendered AI more accessible and efficacious than ever before.
- Availability of Data: The widespread adoption of digital technologies has resulted in a data deluge, supplying the essential raw material for training AI models.
- Competitive Pressure: The increasing adoption of AI by businesses has intensified the pressure on others to adapt or face the risk of obsolescence.
Despite these catalysts, the path to AI adoption is fraught with challenges. The implementation of AI necessitates substantial investment in terms of capital, time, and resources. It also demands a paradigm shift, transitioning from a perception of AI as a tool for automation to a recognition of AI as a strategic asset.
Furthermore, AI is not a panacea for all business challenges. It is a tool that, when judiciously employed, can yield substantial benefits. However, it also has inherent limitations and risks that necessitate careful management.
Perspectives: Followers and trailblazers
The strategic decision to adopt AI in an organisation necessitates a clear understanding of the path being pursued. Is the organisation merely jumping on the AI bandwagon, or is it trailblazing a unique path with AI? Here, we delve into the key distinctions between these two approaches and provide operational, people, and technology-grounded questions that decision-makers can ask to discern their path.
Jumping on the bandwagon
Organizations that jump on the bandwagon typically adopt AI because it's the current trend, often driven by a desire to keep pace with competitors. This approach risks a lack of strategic alignment with business objectives and can lead to inefficient use of resources and poor implementation. The focus is often on immediate gains rather than long-term strategic benefits.
Questions to consider:
- Operations: Are we adopting AI technologies primarily because our competitors are, or because we see a clear operational need for them?
- People: Is our team equipped with the necessary skills and training to implement and manage the AI technologies we are considering?
- Technology: Are we investing in AI technologies that align with our current technological infrastructure and capabilities?
Trailblazing with AI
Trailblazing organizations have a clear vision of how AI can support their strategic objectives. They adopt AI to drive innovation and create value, choosing AI technologies that align with their business needs and capabilities. The focus is on how AI can drive long-term growth and transformation.
Questions to consider:
- Operations: How will the adoption of AI technologies improve our operational efficiency and effectiveness? How will they help us meet our strategic objectives?
- People: Do we have a culture of innovation and learning that supports the adoption of new technologies? Is our team ready and willing to adapt to the changes brought about by AI?
- Technology: Do the AI technologies we are considering align with our technological strategy? Are they scalable and adaptable to future needs and changes?

History of AI readiness assessments
AI readiness assessments have evolved over time, reflecting the growing complexity and diversity of AI technologies and their applications. Several models have been developed to guide organizations in assessing their readiness for AI adoption. Here, we explore some of these models, their common aspects, and their unique features.
Models for AI Readiness Assessment
- Gartner's AI Readiness Model: This model focuses on three key areas: data and infrastructure readiness, skills and leadership readiness, and business alignment. It provides a comprehensive framework for assessing an organization's readiness to implement AI technologies.
- Microsoft's AI Maturity Model: This model assesses AI readiness in terms of four dimensions: strategy, culture, capabilities, and operations. It helps organizations understand their current state of AI maturity and identify areas for improvement.
- MIT Sloan's AI Readiness Model: This model emphasizes the importance of leadership and culture in AI readiness. It assesses readiness in terms of strategy, data, technology, process, and talent.
- McKinsey's AI Readiness Model: McKinsey's model focuses on five key dimensions: strategy, data, technology, organization, and capabilities. It provides a detailed framework for assessing both technical and organizational readiness for AI.
- World Economic Forum's AI Readiness Model: This model, developed in collaboration with McKinsey, assesses AI readiness at the country level. It considers a range of factors, including human capital, research and development, infrastructure, public services, and public policy.
Convergence among the models
Despite their differences, these models share several common aspects:
- Strategy: All models emphasize the importance of having a clear AI strategy that aligns with the organization's overall business objectives.
- Data: The availability and quality of data is a common focus area. Data is the fuel for AI, and its readiness is a critical factor in AI adoption.
- Technology: The readiness of the organization's technological infrastructure to support AI technologies is another common aspect.
- People and Skills: The models recognize the importance of having the necessary skills within the organization to implement and manage AI technologies.
Divergence among the models
While there are common aspects, the models also diverge in certain areas:
- Leadership and Culture: The emphasis on leadership and culture varies among the models. While the MIT Sloan model places a strong emphasis on these aspects, others may focus more on technical factors.
- Business Alignment: The Gartner and McKinsey models uniquely emphasize the importance of aligning AI technologies with business needs and objectives.
- Capabilities and Operations: The Microsoft and McKinsey models include a specific focus on capabilities and operations, assessing the organization's ability to implement AI technologies and integrate them into its operations.
- Public Services and Policy: The World Economic Forum's model uniquely considers public services and policy, reflecting its focus on AI readiness at the country level.
AI readiness assessments have come a long way, evolving from simple checklists to comprehensive models that consider a wide range of factors. Regardless of the model used, the goal is the same: to help organizations assess their readiness for AI adoption and guide them on their AI journey.
RapidCanvas AI readiness assessment framework
A practical, easy-to-understand model to gauge AI readiness is paramount. The RapidCanvas AI Readiness Assessment Framework is crafted to fulfill this requirement, offering a streamlined, action-oriented approach that is particularly beneficial for businesses contemplating AI solutions.
This framework is not just about simplicity and pragmatism; it's about momentum. It's designed to help organizations not only assess their current state but also to identify actionable steps towards AI adoption. It's less about creating ideal conditions and more about understanding how to start the AI journey effectively, regardless of where you are starting from.
The RapidCanvas framework is structured around three key elements: data, decisions, and operations. These elements focus on the most pressing considerations for businesses adopting AI solutions, and let you focus on getting a jump start.
Data
Data is the lifeblood of AI. The quality, quantity, and accessibility of data directly impact the effectiveness of AI solutions. This element involves assessing the organization's data readiness for AI.
Questions to consider include:
- Do we have the necessary data to support our AI initiatives?
- Is our data clean, reliable, and accessible?
- How can we leverage our data to maximize the benefits of AI?
Decisions
AI has the potential to significantly enhance decision-making processes, providing insights that can lead to more informed, data-driven decisions. This element involves considering how AI can enhance the organization's decision-making processes.
Questions to consider include:
- Which decision-making processes could be improved with AI insights?
- How can AI help us make more data-driven decisions?
- What are the potential impacts on efficiency and effectiveness?
Operations
The integration of AI into the organization's operations can lead to increased efficiency and effectiveness. However, it also requires changes to processes and workflows. This element involves considering how AI will be integrated into the organization's operational processes.
Questions to consider include:
- How will AI technologies be integrated into our existing operations?
- What changes will we need to make to our processes and workflows?
- How will we manage the operational risks associated with AI?
Environment
Several key aspects in the business environment signal the urgency to embrace AI. The questions here serve as guideposts to assess the need for AI adoption and unlock the transformative power it offers.
- Intensifying competition:
- Are competitors leveraging AI to gain advantages in customer experience, operational efficiency, or product innovation?
- How does our organization compare to competitors in terms of AI adoption and its impact on key business areas?
- Technological advancements outpacing progress:
- Are technological advancements, including AI, outpacing our organization's progress?
- Are we falling behind in adopting emerging technologies, particularly in the realm of AI?
- How can we incorporate AI to seize opportunities, drive innovation, and maintain relevance?
- Stagnation and innovation gap:
- Is our organization complacent, resistant to change, and hesitant to explore new ideas?
- Do we find ourselves stuck in a state of stagnation, lacking innovation and growth?
- How can AI serve as a catalyst to reignite innovation, unlock untapped potential, and foster a culture of continuous improvement?
Success with AI: Milestones and key indicators
The adoption of AI is a journey, not a destination. Organizations must have a clear understanding of what success looks like in the context of their AI initiatives. This understanding helps build momentum, establish goals, and measure progress.
Milestones
The following are key milestones that organizations can use to measure their success with AI:
- Proof of Concept: Successful AI initiatives often begin with a small, focused proof of concept that demonstrates the potential value of AI to the organization. This proof of concept should be designed to address a specific business problem or opportunity.
- Pilot Program: Building on the proof of concept, organizations can move to a pilot program that tests the AI solution in a more extensive operational environment. The pilot program should be designed to provide data that can be used to refine the AI solution and identify any issues that might arise.
- Full-Scale Implementation: Once the pilot program has been successfully completed, organizations can move to full-scale implementation. This phase involves integrating the AI solution into the organization's operations and workflows, training personnel, and ensuring that the solution is delivering the intended benefits.
- Continuous Improvement: Successful AI initiatives are never truly complete. Organizations must continuously refine and optimize their AI solutions to ensure that they remain aligned with business objectives and deliver value over the long term.
Key indicators
The following are key indicators that organizations can use to measure their progress towards success with AI:
- Business Impact: The impact of AI solutions on business operations, customer experience, and revenue growth is a critical indicator of success. Organizations should measure the impact of AI in terms of key performance indicators (KPIs) that align with business objectives.
- Data Quality and Accessibility: The availability and quality of data are critical factors in the success of AI initiatives. Organizations should measure their progress in improving data quality and accessibility and ensure that their AI solutions are built on a solid data foundation.
- Operational Efficiency: AI solutions should improve operational efficiency, reducing costs and increasing productivity. Organizations should measure the impact of AI solutions on operational efficiency and identify areas for improvement.
- Employee Adoption: Successful AI solutions require employee adoption and engagement. Organizations should measure employee adoption rates and ensure that employees have the necessary skills and training to use AI solutions effectively.
To succeed with AI requires firstly a clear reference to what success looks like, as well as a set of milestones and key indicators that guide progress. Organizations that adopt AI strategically, aligning with business objectives, and measuring progress towards key milestones and indicators, will be well-positioned to reap the benefits of this transformative technology.
So, you are ready! What next?
You have assessed your organization's readiness for AI adoption and identified the potential applications of AI that align with your strategic objectives. What should be your next steps? Here are some considerations to keep in mind:
Hiring a Data Scientist
One option is to hire a data scientist to develop and implement AI solutions. Data scientists possess the technical expertise necessary to build and train AI models, as well as the ability to analyze and interpret the data produced by these models. However, hiring a data scientist may not be feasible for all organizations, given the high demand and cost associated with this skillset.
Hiring a Service Provider
Another option is to engage a service provider to build and implement AI solutions. Service providers can provide access to a range of expertise, from data science to software development, and can handle the end-to-end process of AI implementation. However, working with a service provider can be costly and may not align with the organization's internal capabilities and culture.
Opting for a No-Code Platform
A third option is to opt for a no-code platform to build and implement AI solutions. No-code platforms enable individuals without technical programming skills to build AI solutions using drag-and-drop interfaces and pre-built templates. This approach can be cost-effective and can enable organizations to leverage the expertise of non-technical employees.
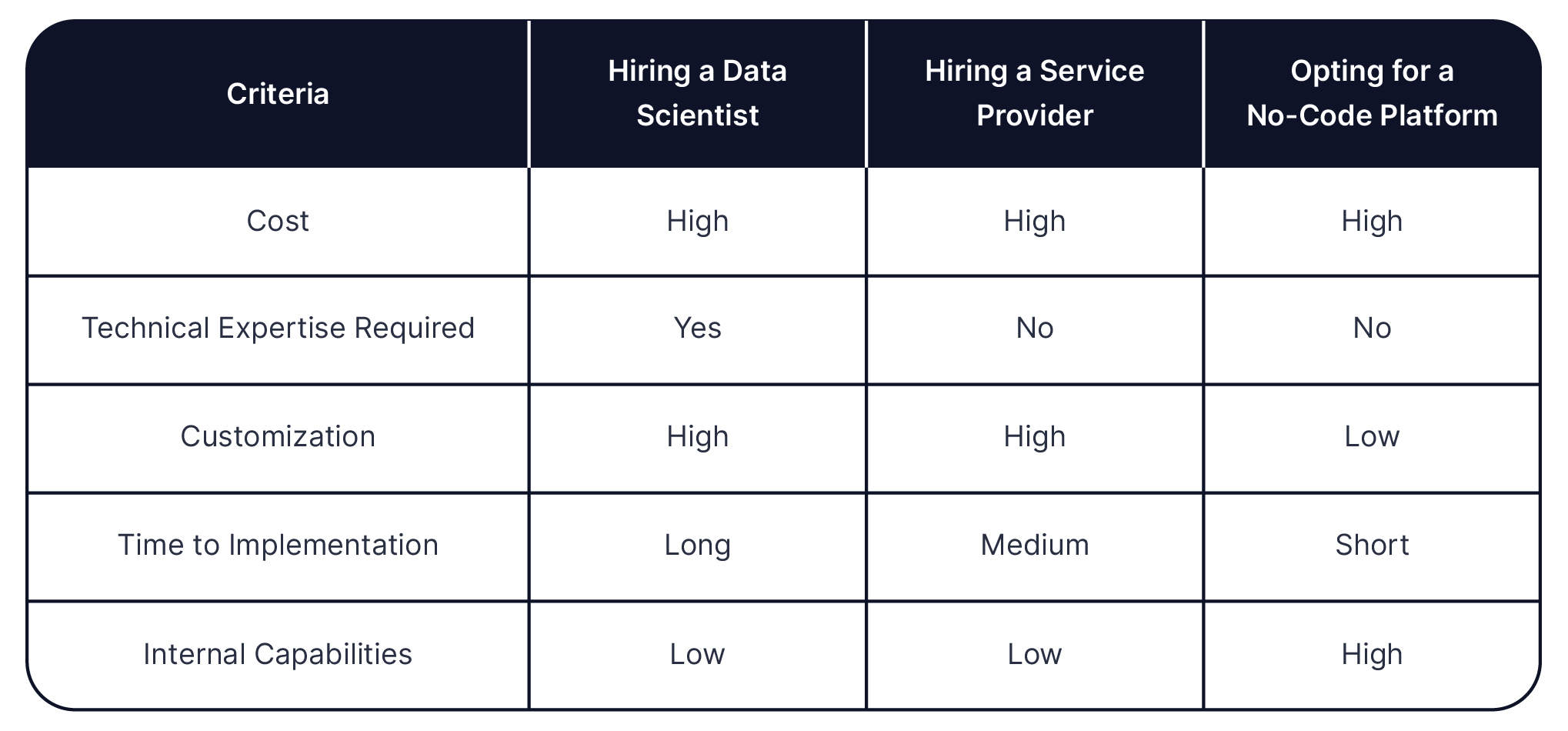
No-code platforms offer several benefits:
- Cost-Effective: No-code platforms can be significantly less expensive than hiring a data scientist or engaging a service provider.
- Ease of Use: No-code platforms enable individuals without technical programming skills to build AI solutions.
- Internal Capabilities: No-code platforms enable organizations to leverage the expertise of non-technical employees and build internal AI capabilities.
- Time to Implementation: No-code platforms can enable faster time to implementation than other options.
In conclusion, there are several options available for organizations looking to adopt AI. While hiring a data scientist or engaging a service provider may be appropriate for some organizations, opting for a no-code platform can provide a cost-effective, easy-to-use, and scalable option for building and implementing AI solutions.
Why no-code AutoAI is the future
The adoption of AI has been hindered by the perception that it is complex and requires significant technical expertise. However, recent developments in AutoAI have made AI more accessible than ever before, enabling individuals without technical programming skills to build and implement AI solutions.
AutoAI involves the use of automated machine learning (AutoML) to build and train AI models. AutoML is a set of algorithms that automatically discover the best machine learning models for a given dataset, without requiring human intervention. This approach can significantly reduce the time and effort required to build and train AI models, enabling organizations to focus on using AI to drive value and innovation.
No-code AutoAI platforms provide a streamlined, easy-to-use approach to building and deploying AI solutions. These platforms enable individuals without technical programming skills to build AI solutions using drag-and-drop interfaces and pre-built templates. They can significantly reduce the costs associated with AI adoption and enable organizations to leverage the expertise of non-technical employees.
Benefits of no-code AutoAI
No-code AutoAI offers several benefits:
Ease of Use
No-code AutoAI platforms enable individuals without technical programming skills to build and deploy AI solutions. This approach can democratize AI and enable organizations to leverage the expertise of non-technical employees.
Cost-Effective
No-code AutoAI platforms can significantly reduce the costs associated with AI adoption. They eliminate the need for costly data scientists and enable organizations to build and deploy AI solutions using existing resources.
Rapid Deployment
No-code AutoAI platforms can enable organizations to rapidly deploy AI solutions. They can significantly reduce the time and effort required to build and train AI models, enabling organizations to focus on using AI to drive value and innovation.
Scalability
No-code AutoAI platforms can enable organizations to scale their AI solutions rapidly. They can easily integrate with existing systems and processes, enabling organizations to leverage their AI solutions across the enterprise.
Flip the game: Find value before you choose to invest
The adoption of AI can transform businesses, driving growth and innovation. However, it is not a panacea for all business challenges. Investment in AI requires careful consideration and strategic planning. It involves assessing the organization's readiness for AI adoption, identifying potential applications of AI that align with strategic objectives, and selecting the right approach to implementing AI.
Here are five key points to consider when investing in AI:
Ask for a prototype
Before investing in AI, ask for a prototype that demonstrates the potential value of AI to the organization. This prototype should be designed to address a specific business problem or opportunity. It should demonstrate how AI can drive efficiency, innovation, and growth.
See value
Assess the value of the prototype, considering how it aligns with the organization's business objectives. Ensure that the prototype delivers tangible benefits, such as increased operational efficiency, improved customer experience, or revenue growth. Identify key performance indicators (KPIs) that align with business objectives and use them to measure the impact of the prototype.
Assess fit
Consider how the prototype fits with the organization's technological infrastructure, capabilities, and culture. Assess the organization's readiness for AI adoption and identify any areas for improvement. Ensure that the prototype aligns with the organization's technological strategy and can be integrated into existing systems and processes.
Invest
Once the prototype has been successfully assessed, invest in AI, choosing the approach that best aligns with the organization's objectives, capabilities, and culture. This may involve hiring a data scientist, engaging a service provider, or opting for a no-code platform. Ensure that the investment is aligned with the organization's budget and risk tolerance.
Scale
Finally, scale the AI solution, integrating it into the organization's operations and workflows, training personnel, and ensuring that the solution is delivering the intended benefits. Continuously refine and optimize the AI solution to ensure that it remains aligned with business objectives and delivers value over the long term.
Shed the inertia and inhibitions and take the first step towards AI adoption. By asking for a prototype, assessing the value, assessing fit, investing, and scaling, businesses can unlock the transformative power of AI to drive growth and innovation.